Behind the buzzword: Data quality
Why seemingly no one in the advertising ecosystem is rightly incentivized to tackle this
Third-party data rests at the backbone of the US data-driven marketing ecosystem. The sector has enjoyed robust, frequently double-digit annual growth especially since DSPs and DMPs popularized the relatively easy collection, packaging, and transaction of digitally-originating third-party data sets over the past decade+. According to the latest IAB & Winterberry Group annual State of Data report, 2019 saw a slowing 6.1% increase over 2018 (the 2017-2018 growth rate previously clocked in at 15.7%). In money terms that translates to a nicely-sized $11.9 billion annual market for third-party data; on top of this, another $7.8 billion is spent annually on audience data activation solutions that enable the use of these data sets for analysis and targeting.
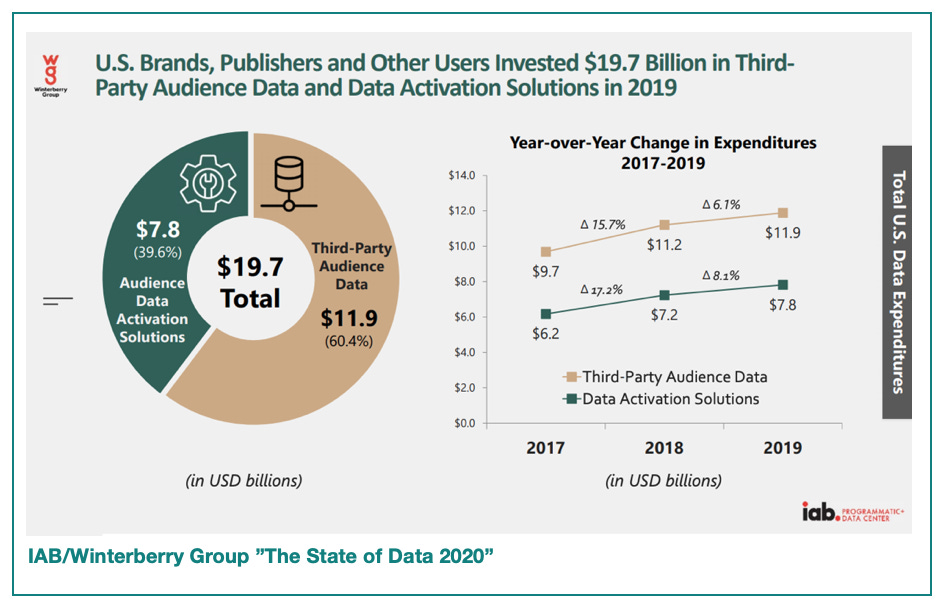
The third-party data is experiencing a variant of the type of spend shift we’ve seen between traditional and emerging channels (like linear TV and digital): while overall spend is growing, the amount spent on offline (terrestrial) channels like direct mail is shrinking year over year; at the same time the amount spent on digital solutions has been steadily increasing, lessening the initial spend gap between the two. Uncertainty around the demise of third-party cookies, the shift in interest towards first party data, looming data regulation, and questions around data quality are contributing to slowing down the rate of increase of digital data investment (from an astronomical 36.6% in the previous year to a still-high 13.8% in 2019). While the trend is for those two categories to equalize and eventually flip over the coming years, a third distinct category is emerging to grab spend share from both: advanced TV. While we’d argue that advanced TV should likely count under the digital umbrella we can certainly understand why it warrants a separate category: the budget for it has to come from somewhere, and increasingly advanced/connected TV represents a budget line-item of its own, separate from digital video and linear upfront & scatter. In 2019 data-related spending on advanced television represented 5% of the US third-party data market.
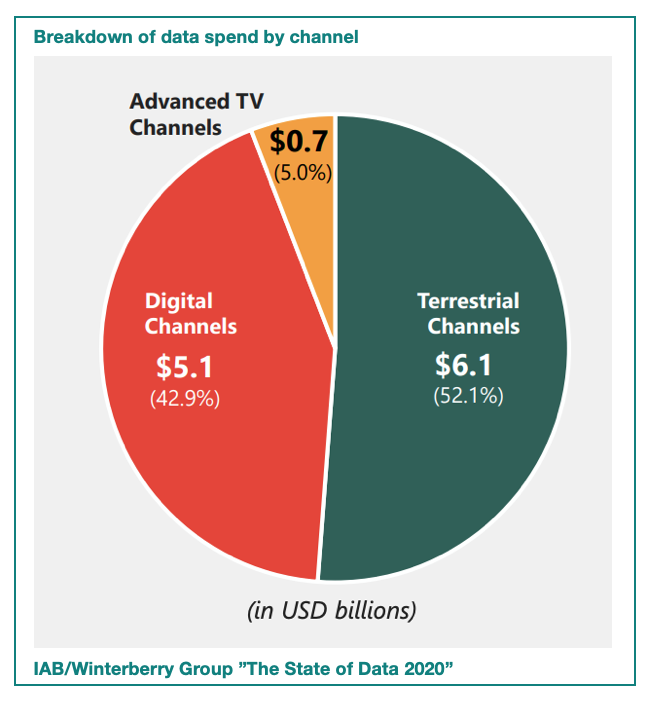
Yet if you browse most publicly available third-party data sets on your data/targeting platform of choice or their corresponding data lexicons (like this old one from Oracle Data Cloud) it’s relatively easy to get lost in a sea of data providers who describe their segments in similar if not nearly identical ways. The bulk of third-party digital data spend is on demographic data: the famous ZAG (zip/age/gender) trio that accounted for 37% of all data sales in 2019 (the other major groups are behavioral at 23.5%, transactional at 24.4%, and location at 15.1% per the IAB/Winterberry data study).
Therein lies the rub: for 75% of the market there’s practically no agreement on what constitutes data quality, what differentiates a strong data signal from a weak one, or what should be qualifying criteria for a particular segment at all. The third-party data marketplace is opaque by design.
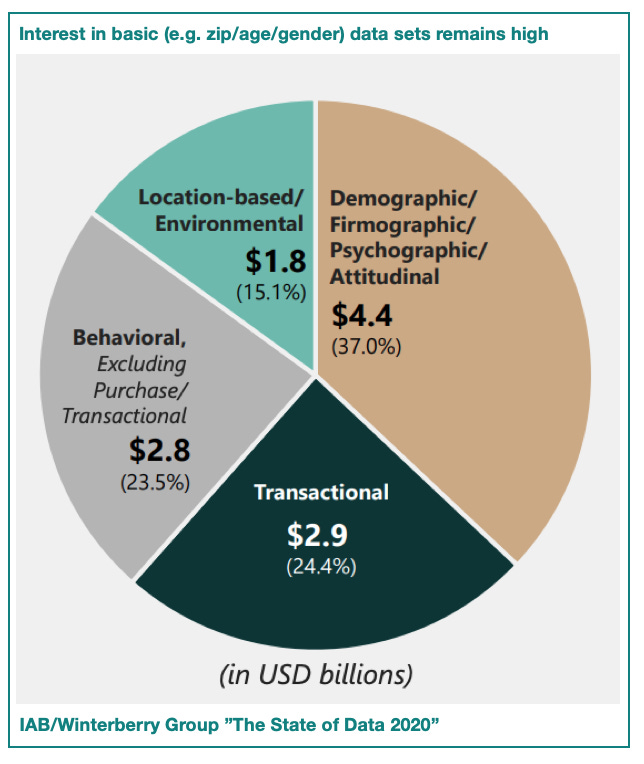
How do data quality issues manifest in practice? It’s a nuanced challenge and frequently hard to observe and diagnose. Consider the following real-life examples for some color:
A well-known hospitality group was looking to advertise special packages to folks approaching marquee birthdays. They selected a reputable provider and purchased age, gender, and zipcode data. In their campaign analysis they noticed that they were serving a lot of ads to people about to turn 50, 55, and 60 years of age -- significantly more than there were such people in the regions of interest according to census data. Subsequent prodding and analysis identified that while the reputable data provider specified they are selling ‘year of birth’ age, some of the publishers they were working with were grouping age information into ranges and rounding to the closest 5-year increment, thus polluting the underlying data set. This went undetected by the reputable data provider; how many other brands and buyers used that same data set without discovering the discrepancy?
A niche clothing and apparel company was prepping a campaign aimed at women shoppers living in rural areas. They purchased a gender data set from a reputable provider and assumed that it would be accurate. The data provider used a proprietary name-to-gender translator to infer gender and collected this data mainly from a variety of forms like appliance warranty registrations, assorted sweepstakes, and similar fairly low-quality fare. The name-to-gender algorithm handled Mike and Nancy well; it (perhaps predictably) didn’t know what to do with Asian, South-Asian, Pacific, and other non-white ethnic group names: precisely the groups that this brand wanted to reach. The client in this case was swayed by the data provider’s claims at gender accuracy without digging deeper into the mechanics of subsets that were particularly relevant to them (and ended up wasting money).
A luxury auto manufacturer wanted to target people who are highly likely to purchase a luxury vehicle in the next 45 days across 3 Northeastern states. Targeting platforms their teams had access to returned an estimated segment size in the 10s of millions: an orders of magnitude larger data set than actual auto sales (especially in a relatively small and well-defined region) would support.
This takes us back to the original sin of digitally-originating third-party data: while there is at least some expectation of veracity in predominantly offline data sets, in digital data sets we have little recourse then to take someone’s word for what their data set really represents.
Any publisher can create their own interpretation of what constitutes a data point - especially for interest and intent segments. Clicked on a random article about vegan food? Congrats, you’re now a vegan (for advertising purposes)! Looked at that one Porsche while researching the trade-in value of your family’s car? You can look forward to more Porsches, Bentleys, Audis, Beemers, Range Rovers, etc in your daily internet experience (the perks of qualifying for a luxury auto intender segment).
Why hasn’t data quality been addressed in a more systematic way? Chalk this one up to misaligned incentives, like probably 99% of other advertising problems. On the buy side, many agencies interpret the choices they make with client’s budgets as their unique intellectual property/secret sauce. What that very often translates to is that a campaign trafficker will buy the cheapest possible version of a data point, track performance, and if it’s anything less than catastrophic likely won’t adjust (especially in cases where any difference in data costs goes back to the agency as margin). On the brand side, there’s an endless list of data providers to choose from and learn about. Unless brands have strict criteria on data quality markers they want to meet (and very few do), the squeakiest wheel - and not necessarily the best - often wins. This leaves us with the tech platforms in the middle: many are both aggregators and sellers of data, and they are incentivized to increase sales - and not necessarily improve the product (after all, if buyers aren’t complaining what’s to improve?). Those that are just data marketplaces largely prefer to stay neutral and leave the choice of which data set to buy to the actual buyer. Several have rolled out their own data scoring to support buyers on platform -- a welcome measure that can at least help directionally, but raises its own questions about the veracity of scoring algorithms. Just about the only ones largely immune to these considerations are walled gardens who simply no longer need third party data at all.
Ok. How do we fix this? As tempting as it is to go all ‘AI/ML’ and ‘algorithmic segment scoring’ here, the quickest solution might be far more low-tech and easier to implement widely. The IAB Tech Lab, along with member companies and several other industry organizations, has been working on a common data transparency framework. The model is of nutritional labels on food, but for data: a simple way of flagging a data point’s origin, what it’s meant to represented, where/how it was constructed, and what are its proverbial ingredients hailing from (how was the data sourced/collected). Delightfully simple and straightforward that it makes one wonder why this level of transparency wasn’t the starting point and required by buyers from the get-go.
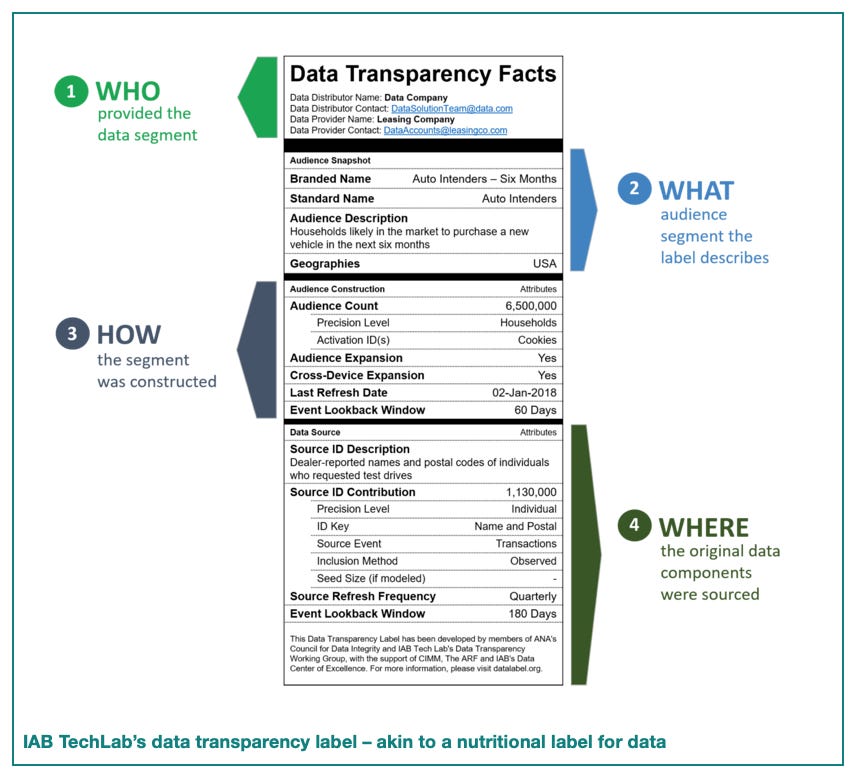
Last week on The Big Story podcast AdExchanger’s managing editor Ryan Joe asked our co-founder (and Chief Tweeter) Ana Milicevic if third party data gets a bad rap or if it's universally bad. It’s really a missed opportunity more than anything else: a chance to define the basic components of what would make a higher-quality data set. As we as an industry transition to first-party data, data quality will be a recurring challenge for publishers and buyers alike: what distinguishes one publisher’s data set from another’s? Here’s hoping the industry won’t repeat the same mistakes with third-party data all over again, like some extremely bad version of Groundhog Day.
One question:
Now that we (finally) have somewhat of an industry-wide consensus on the basic definitions of data quality, momentum on who’s driving this issue has visibly shifted from the open internet. This begs the question of who can solve a complex issue like data quality and transparency and faster: the open internet (through transparency initiatives like the Data Label), regulators (through data legislation like GDPR, CCPA/CPRA and other regional/country-specific versions), or walled gardens (through commanding an ever-increasing portion of ad spend)?
Dig deeper:
Digiday’s excellent ‘WTF’ series explaining the data label and other transparency efforts
IAB/Winterberry State of Data reports
Enjoyed this piece? Share it, like it, and send us comments (you can reply to this email).
Who we are: Sparrow Advisers
We’re a results oriented management consultancy bringing deep operational expertise to solve strategic and tactical objectives of companies in and around the ad tech and mar tech space.
Our unique perspective rooted deeply in AdTech, MarTech, SaaS, media, entertainment, commerce, software, technology, and services allows us to accelerate your business from strategy to day-to-day execution.
Founded in 2015 by Ana and Maja Milicevic, principals & industry veterans who combined their product, strategy, sales, marketing, and company scaling chops and built the type of consultancy they wish existed when they were in operational roles at industry-leading adtech, martech, and software companies. Now a global team, Sparrow Advisers help solve the most pressing commercial challenges and connect all the necessary dots across people, process, and technology to simplify paths to revenue from strategic vision down to execution. We believe that expertise with fast-changing, emerging technologies at the crossroads of media, technology, creativity, innovation, and commerce are a differentiator and that every company should have access to wise Sherpas who’ve solved complex cross-sectional problems before. Contact us here.